What is Yield Optimization for Podcasting?
The textbook definitions of Yield Optimization hide the complexity of the challenge.
I recently had someone ask me to explain, in very simple language, what we mean by Yield Optimization at Flightpath.
This is not the first time I’ve been asked this, and I found myself struggling to answer clearly and simply. I could turn to a textbook definition:
The process aimed at ad campaign optimization, increasing its effectiveness and output that is achieved by influencing consumer’s behavior in a profit-maximizing manner and better inventory management. (source: SmartyAds)
But this downplays the significant technical and integration hurdles our industry faces. I expect that the person asking wants to know how we think about yield and what our promise is to our clients.
Our promise is easy to state: to reduce make goods, lower unsold inventory, and increase direct sold sell-through by at least 5 points on our clients’ largest shows.
But achieving this promise is anything but simple and quickly gets us to the heart of the yield optimization problem. I’ve broken our approach to yield optimization into three distinct but inter-related arenas. I believe that significant gains in each of these arenas is a prerequisite for being able to fully deliver on our promise.
Arena One: Clean and Trusted Data
Another way to state this is, “to make the invisible visible and the unreliable reliable.”
Let’s start with fill-rates. Publishers have limited or no visibility into their fill-rates, let alone trusted unsold inventory numbers. Relying instead on back-of-a-napkin estimates.
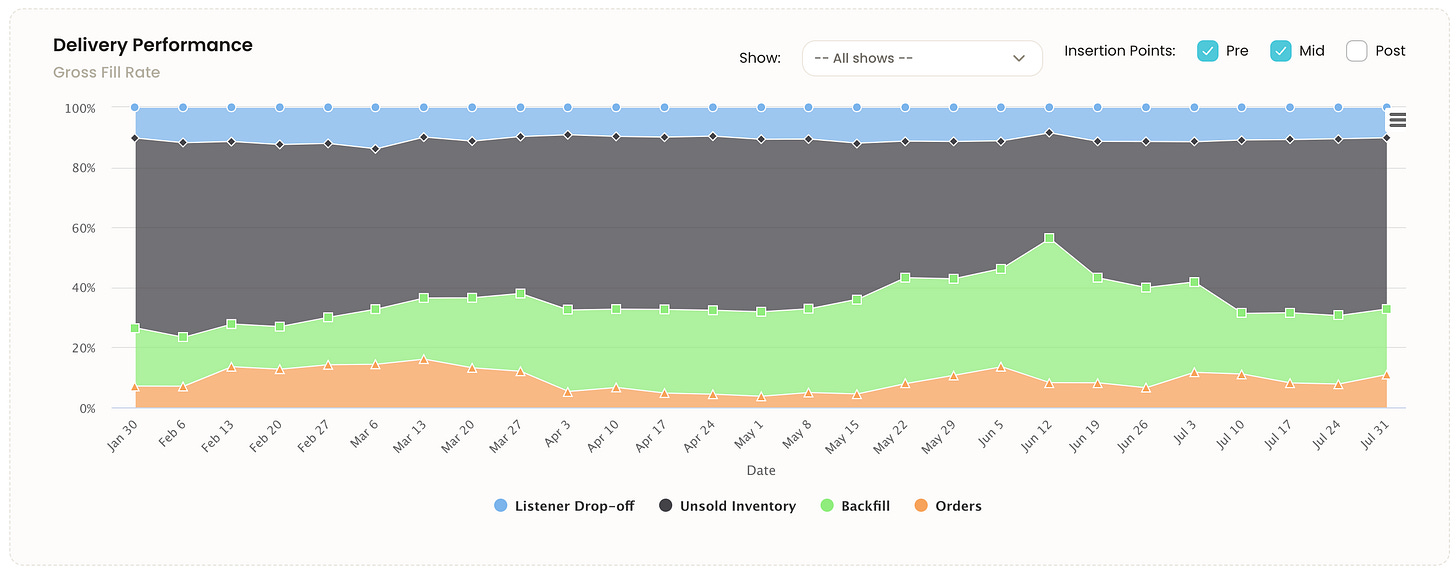
The Flightpath platform exposes network-wide and show-level fill-rate information. For many of our clients, this is the first time they are able to easily see this information, let alone the percentage of downloads where impressions can’t be delivered. This is the missing 10% to 15% many of us in ad operations felt and suspected, but could never fully understand or prove. And it explains why everyone’s estimates based on downloads were inaccurate and causing their sales teams to oversell inventory.
This is just one examples of how yield is dependent on making the invisible visible.
We also need clean data.
Data cleaning is the process of aligning data between sources and flagging, removing or updating data to better match expected reality.
Customers who use a Sales Force Automation platform often have a method for flighting sold orders into their DAI podcast hosting platform. This process can even replicate advertisers and the agencies that represent them into the DAI host. The idea being that this will take care of the trafficking of orders. Everything is done for your team. Sales places the order and now it’s in the podcast host, just waiting on creative.
The reality is anything but.
Many of these trafficked orders are missing key data such as insertion points, or they are applied to an out-of-date agency. Anyone who’s been a publisher in this space has likely sold BetterHelp through at least three agencies, if not five or six. And while publishers make sure to update their Sales Force Automation platform or sales spreadsheets, this rarely makes its way into the DAI podcast host.
If we want to identify opportunities to sell unsold inventory in the future, we need to be 100% confident in what was sold and to whom. All the sources need to align or we risk wasting endless person hours untangling issues between delivery and billing.
Finally, many of our clients represent third-party shows for sponsorship which means they now have to manage the producers for each of these shows. And here enters one of the trickiest problems in yield: reliable future episode release data.
There are a number of behaviours that producers can (and will) exhibit that directly and negatively impact sales and yield, often unbeknownst to the network or publisher:
They go on vacation without telling anyone and schedule re-runs that most listeners have already heard
Suddenly forget to put mid-roll insertion points into their episodes, causing all your orders to cascade into trouble
Drop someone else’s episode into their feed as a promotion (far less people hit play on it)
Change their season release and forget to tell anyone
As the number of producers in a network climbs, so does the variance in the reliability of the data these producers enter (or don’t enter) into the podcast DAI host. This can have one of the most significant impacts to future downloads, almost always in the negative direction, causing campaigns to under-perform and subsequent make goods to trigger a domino effect across every other buy touching the impacted show(s).
Some networks have responded by taking producer functions in-house, with full-time-employees responsible for checking every episode or even uploading files for the producers. But when you look at the complexity of entering show notes, setting insertion points and configuring every episode with release dates that get delayed or pushed, this quickly becomes a scaling issue for any company. We generally don’t recommend this.
Instead, what if we could use intelligent software systems to better understand, predict, and catch issues across all these producers and their shows? And even predict expected producer behaviour in many cases. This would be far more scalable and likely more effective as well.
These are a just a few of the areas where data the validity of the inputs is crucial to understanding, predicting, and managing yield.
Arena Two: Predictive Performance
At Flightpath, we don’t deliver ads, nor do we deliver podcasts. We predict future performance and availability. This means we work with our clients and their DAI podcast hosts and Sales Force Automation (SFA) partners to understand what was sold, what has been flighted, and how everything is performing.
Developing Flightpath was a constant dance between improving our prediction algorithms while also solving for issues of incomplete and unreliable data.
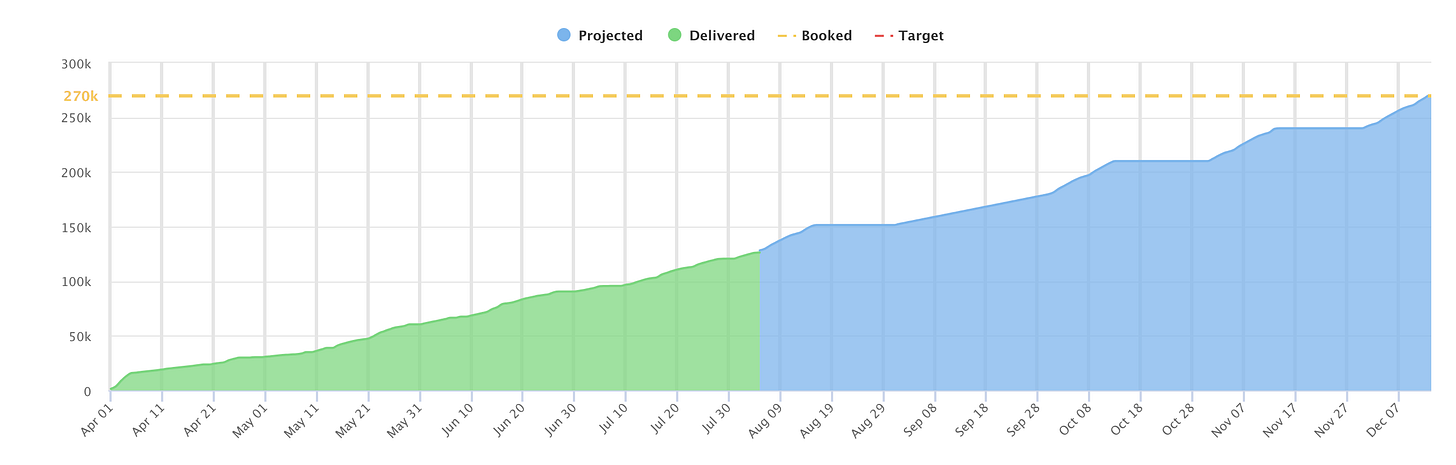
The result is our latest Precog engine and platform which is able to predict order and campaign performance twelve months into the future with what we believe to be the best accuracy to-date in the industry.
Predicting performance gives some pretty amazing tools to your ad operations, sales, and finance teams. They can better identify issues before the campaign has even launched, improve their financial predictions, eliminate barriers to scaling their businesses, and deploy their people where the problems are, versus trying to manage thousands of orders every day in a fury of spreadsheets and data re-entry.
This drives better buyer relationships, lower make goods, and less stress for everyone involved.
Our latest achievement in this arena was to give the sales and sales support teams access to real-time, trusted unsold inventory.
My experience as a buyer in the podcast space made it pretty apparent that publishers of all sizes were struggling to turnaround availability. It was not uncommon for a publishers to take weeks to respond with the impressions they had available. And worse, these numbers were often anything but accurate, leading to frustration as a buyer and a growing lack of trust of the publishers.
This next bit can not be overstated. Giving sales teams real-time access to trusted availability is one of the greatest levers to increase yield and sell-through. Closing deals quickly and then delivering as promised is a very effective way to turn first-time buyers into repeat customers.
This is because publishers and networks are not selling hard goods; they are making promises. They are promising a buyer an amount of inventory on a specifics show (or set of shows) within a specific time period in the future. And further, with specific attributes such as: US-only and frequency capped at 3 listens in any 7 day period.
Breaking these promises is one of the biggest challenges that the podcast industry faces. And it is directly tied to yield and trusted sales availability.
Arena Three: Automation and Consumer Behaviour
This is the arena we are just beginning to play within.
Our 1.2 release will offer new pacing algorithms that manage orders within their respective DAI platforms to maximize performance and fill-rates. And we are excited to unveil new pacing options that buyers and ad ops teams are asking for.
And there are a lot of areas where automation can allow us to leverage the insights we have to drive better yield and sell-through for the publisher. But this is just the beginning.
Our clients need to be able to optimize performance around the KPIs that align with the buyer’s campaign goals. This requires different decisions based on new signals. Signals that need to be integrated, cleaned, trusted and predicted. Sound familiar?
The arenas will be the same, but the outcome will finally be the textbook definition of yield optimization.
In summary…
I am incredibly proud of our accomplishments in each of the above arenas. We have achieved visibility into real-time, order-placeable unsold inventory 9 months into the future. We have flight and campaign predictions up to a year in the future. And we’ve deployed a growing number of fixes required to clean up the data across the sales and operations lifecycle, not to mention our ad ops alerts, creative workflow management, and other solutions.
I’m most proud of our impact on direct sold sell-through. A day doesn’t go by without someone touting programmatic marketplaces as the solution to the unsold inventory problem. And I completely agree that programmatic will play a role, but with so many marketplaces bringing in $3 to $7 CPM along with low fill rates, I am proud to be helping our clients drive increases in direct sold inventory where they can achieve CPM rates more in the $20 to $25 range.
There is still work to be done, but I am excited to see Flightpath play a role in the future of yield for the creator-based economy.